涨知识!用逻辑规则进行机器学习
今日不肯埋头,明日何以抬头!每日一句努力自己的话哈哈~哈喽,今天我将给大家带来一篇《涨知识!用逻辑规则进行机器学习》,主要内容是讲解等等,感兴趣的朋友可以收藏或者有更好的建议在评论提出,我都会认真看的!大家一起进步,一起学习!
Skope-rules使用树模型生成规则候选项。首先建立一些决策树,并将从根节点到内部节点或叶子节点的路径视为规则候选项。然后通过一些预定义的标准(如精确度和召回率)对这些候选规则进行过滤。只有那些精确度和召回率高于其阈值的才会被保留。最后,应用相似性过滤来选择具有足够多样性的规则。一般情况下,应用Skope-rules来学习每个根本原因的潜在规则。
项目地址:https://github.com/scikit-learn-contrib/skope-rules
- Skope-rules是一个建立在scikit-learn之上的Python机器学习模块,在3条款BSD许可下发布。
- Skope-rules旨在学习逻辑的、可解释的规则,用于 "界定 "目标类别,即高精度地检测该类别的实例。
- Skope-rules是决策树的可解释性和随机森林的建模能力之间的一种权衡。
schema
安装
可以使用 pip 获取最新资源:
pip install skope-rules
快速开始
SkopeRules 可用于描述具有逻辑规则的类:
from sklearn.datasets import load_iris from skrules import SkopeRules dataset = load_iris() feature_names = ['sepal_length', 'sepal_width', 'petal_length', 'petal_width'] clf = SkopeRules(max_depth_duplicatinotallow=2, n_estimators=30, precision_min=0.3, recall_min=0.1, feature_names=feature_names) for idx, species in enumerate(dataset.target_names): X, y = dataset.data, dataset.target clf.fit(X, y == idx) rules = clf.rules_[0:3] print("Rules for iris", species) for rule in rules: print(rule) print() print(20*'=') print()
注意:
如果出现如下错误:
解决方案:
关于 Python 导入错误 : cannot import name 'six' from 'sklearn.externals' ,云朵君在Stack Overflow上找到一个类似的问题:https://stackoverflow.com/questions/61867945/
解决方案如下
import six import sys sys.modules['sklearn.externals.six'] = six import mlrose
亲测有效!
如果使用“score_top_rules”方法,SkopeRules 也可以用作预测器:
from sklearn.datasets import load_boston from sklearn.metrics import precision_recall_curve from matplotlib import pyplot as plt from skrules import SkopeRules dataset = load_boston() clf = SkopeRules(max_depth_duplicatinotallow=None, n_estimators=30, precision_min=0.2, recall_min=0.01, feature_names=dataset.feature_names) X, y = dataset.data, dataset.target > 25 X_train, y_train = X[:len(y)//2], y[:len(y)//2] X_test, y_test = X[len(y)//2:], y[len(y)//2:] clf.fit(X_train, y_train) y_score = clf.score_top_rules(X_test) # Get a risk score for each test example precision, recall, _ = precision_recall_curve(y_test, y_score) plt.plot(recall, precision) plt.xlabel('Recall') plt.ylabel('Precision') plt.title('Precision Recall curve') plt.show()
实战案例
本案例展示了在著名的泰坦尼克号数据集上使用skope-rules。
skope-rules适用情况:
- 解决二分类问题
- 提取可解释的决策规则
本案例分为5个部分
- 导入相关库
- 数据准备
- 模型训练(使用ScopeRules().score_top_rules()方法)
- 解释 "生存规则"(使用SkopeRules().rules_属性)。
- 性能分析(使用SkopeRules.predict_top_rules()方法)。
导入相关库
# Import skope-rules from skrules import SkopeRules # Import librairies import pandas as pd from sklearn.ensemble import GradientBoostingClassifier, RandomForestClassifier from sklearn.model_selection import train_test_split from sklearn.tree import DecisionTreeClassifier import matplotlib.pyplot as plt from sklearn.metrics import roc_curve, precision_recall_curve from matplotlib import cm import numpy as np from sklearn.metrics import confusion_matrix from IPython.display import display # Import Titanic data data = pd.read_csv('../data/titanic-train.csv')
数据准备
# 删除年龄缺失的行 data = data.query('Age == Age') # 为变量Sex创建编码值 data['isFemale'] = (data['Sex'] == 'female') * 1 # 未变量Embarked创建编码值 data = pd.concat( [data, pd.get_dummies(data.loc[:,'Embarked'], dummy_na=False, prefix='Embarked', prefix_sep='_')], axis=1 ) # 删除没有使用的变量 data = data.drop(['Name', 'Ticket', 'Cabin', 'PassengerId', 'Sex', 'Embarked'], axis = 1) # 创建训练及测试集 X_train, X_test, y_train, y_test = train_test_split( data.drop(['Survived'], axis=1), data['Survived'], test_size=0.25, random_state=42) feature_names = X_train.columns print('Column names are: ' + ' '.join(feature_names.tolist())+'.') print('Shape of training set is: ' + str(X_train.shape) + '.')
Column names are: Pclass Age SibSp Parch Fare isFemale Embarked_C Embarked_Q Embarked_S. Shape of training set is: (535, 9).
模型训练
# 训练一个梯度提升分类器,用于基准测试 gradient_boost_clf = GradientBoostingClassifier(random_state=42, n_estimators=30, max_depth = 5) gradient_boost_clf.fit(X_train, y_train) # 训练一个随机森林分类器,用于基准测试 random_forest_clf = RandomForestClassifier(random_state=42, n_estimators=30, max_depth = 5) random_forest_clf.fit(X_train, y_train) # 训练一个决策树分类器,用于基准测试 decision_tree_clf = DecisionTreeClassifier(random_state=42, max_depth = 5) decision_tree_clf.fit(X_train, y_train) # 训练一个 skope-rules-boosting 分类器 skope_rules_clf = SkopeRules(feature_names=feature_names, random_state=42, n_estimators=30, recall_min=0.05, precision_min=0.9, max_samples=0.7, max_depth_duplicatinotallow= 4, max_depth = 5) skope_rules_clf.fit(X_train, y_train) # 计算预测分数 gradient_boost_scoring = gradient_boost_clf.predict_proba(X_test)[:, 1] random_forest_scoring = random_forest_clf.predict_proba(X_test)[:, 1] decision_tree_scoring = decision_tree_clf.predict_proba(X_test)[:, 1] skope_rules_scoring = skope_rules_clf.score_top_rules(X_test)
"生存规则" 的提取
# 获得创建的生存规则的数量 print("用SkopeRules建立了" + str(len(skope_rules_clf.rules_)) + "条规则n") # 打印这些规则 rules_explanations = [ "3岁以下和37岁以下,在头等舱或二等舱的女性。" "3岁以上乘坐头等舱或二等舱,支付超过26欧元的女性。" "坐一等舱或二等舱,支付超过29欧元的女性。" "年龄在39岁以上,在头等舱或二等舱的女性。" ] print('其中表现最好的4条 "泰坦尼克号生存规则" 如下所示:/n') for i_rule, rule in enumerate(skope_rules_clf.rules_[:4]) print(rule[0]) print('->'+rules_explanations[i_rule]+ 'n')
用SkopeRules建立了9条规则。 其中表现最好的4条 "泰坦尼克号生存规则" 如下所示: Age 2.5 and Pclass 0.5 -> 3岁以下和37岁以下,在头等舱或二等舱的女性。 Age > 2.5 and Fare > 26.125 and Pclass 0.5 -> 3岁以上乘坐头等舱或二等舱,支付超过26欧元的女性。 Fare > 29.356250762939453 and Pclass 0.5 -> 坐一等舱或二等舱,支付超过29欧元的女性。 Age > 38.5 and Pclass 0.5 -> 年龄在39岁以上,在头等舱或二等舱的女性。
def compute_y_pred_from_query(X, rule): score = np.zeros(X.shape[0]) X = X.reset_index(drop=True) score[list(X.query(rule).index)] = 1 return(score) def compute_performances_from_y_pred(y_true, y_pred, index_name='default_index'): df = pd.DataFrame(data= { 'precision':[sum(y_true * y_pred)/sum(y_pred)], 'recall':[sum(y_true * y_pred)/sum(y_true)] }, index=[index_name], columns=['precision', 'recall'] ) return(df) def compute_train_test_query_performances(X_train, y_train, X_test, y_test, rule): y_train_pred = compute_y_pred_from_query(X_train, rule) y_test_pred = compute_y_pred_from_query(X_test, rule) performances = None performances = pd.concat([ performances, compute_performances_from_y_pred(y_train, y_train_pred, 'train_set')], axis=0) performances = pd.concat([ performances, compute_performances_from_y_pred(y_test, y_test_pred, 'test_set')], axis=0) return(performances) print('Precision = 0.96 表示规则确定的96%的人是幸存者。') print('Recall = 0.12 表示规则识别的幸存者占幸存者总数的12%n') for i in range(4): print('Rule '+str(i+1)+':') display(compute_train_test_query_performances(X_train, y_train, X_test, y_test, skope_rules_clf.rules_[i][0]) )
Precision = 0.96 表示规则确定的96%的人是幸存者。 Recall = 0.12 表示规则识别的幸存者占幸存者总数的12%。
模型性能检测
def plot_titanic_scores(y_true, scores_with_line=[], scores_with_points=[], labels_with_line=['Gradient Boosting', 'Random Forest', 'Decision Tree'], labels_with_points=['skope-rules']): gradient = np.linspace(0, 1, 10) color_list = [ cm.tab10(x) for x in gradient ] fig, axes = plt.subplots(1, 2, figsize=(12, 5), sharex=True, sharey=True) ax = axes[0] n_line = 0 for i_score, score in enumerate(scores_with_line): n_line = n_line + 1 fpr, tpr, _ = roc_curve(y_true, score) ax.plot(fpr, tpr, linestyle='-.', c=color_list[i_score], lw=1, label=labels_with_line[i_score]) for i_score, score in enumerate(scores_with_points): fpr, tpr, _ = roc_curve(y_true, score) ax.scatter(fpr[:-1], tpr[:-1], c=color_list[n_line + i_score], s=10, label=labels_with_points[i_score]) ax.set_title("ROC", fnotallow=20) ax.set_xlabel('False Positive Rate', fnotallow=18) ax.set_ylabel('True Positive Rate (Recall)', fnotallow=18) ax.legend(loc='lower center', fnotallow=8) ax = axes[1] n_line = 0 for i_score, score in enumerate(scores_with_line): n_line = n_line + 1 precision, recall, _ = precision_recall_curve(y_true, score) ax.step(recall, precision, linestyle='-.', c=color_list[i_score], lw=1, where='post', label=labels_with_line[i_score]) for i_score, score in enumerate(scores_with_points): precision, recall, _ = precision_recall_curve(y_true, score) ax.scatter(recall, precision, c=color_list[n_line + i_score], s=10, label=labels_with_points[i_score]) ax.set_title("Precision-Recall", fnotallow=20) ax.set_xlabel('Recall (True Positive Rate)', fnotallow=18) ax.set_ylabel('Precision', fnotallow=18) ax.legend(loc='lower center', fnotallow=8) plt.show() plot_titanic_scores(y_test, scores_with_line=[gradient_boost_scoring, random_forest_scoring, decision_tree_scoring], scores_with_points=[skope_rules_scoring] )
在ROC曲线上,每个红点对应于激活的规则(来自skope-rules)的数量。例如,最低点是1个规则(最好的)的结果点。第二低点是2条规则结果点,等等。
在准确率-召回率曲线上,同样的点是用不同的坐标轴绘制的。警告:左边的第一个红点(0%召回率,100%精度)对应于0条规则。左边的第二个点是第一个规则,等等。
从这个例子可以得出一些结论。
- skope-rules的表现比决策树好。
- skope-rules的性能与随机森林/梯度提升相似(在这个例子中)。
- 使用4个规则可以获得很好的性能(61%的召回率,94%的精确度)(在这个例子中)。
n_rule_chosen = 4 y_pred = skope_rules_clf.predict_top_rules(X_test, n_rule_chosen) print('The performances reached with '+str(n_rule_chosen)+' discovered rules are the following:') compute_performances_from_y_pred(y_test, y_pred, 'test_set')
predict_top_rules(new_data, n_r)方法用来计算对new_data的预测,其中有前n_r条skope-rules规则。
今天关于《涨知识!用逻辑规则进行机器学习》的内容介绍就到此结束,如果有什么疑问或者建议,可以在golang学习网公众号下多多回复交流;文中若有不正之处,也希望回复留言以告知!

- 上一篇
- 预见2032:探索人工智能与开发者的下一个十年
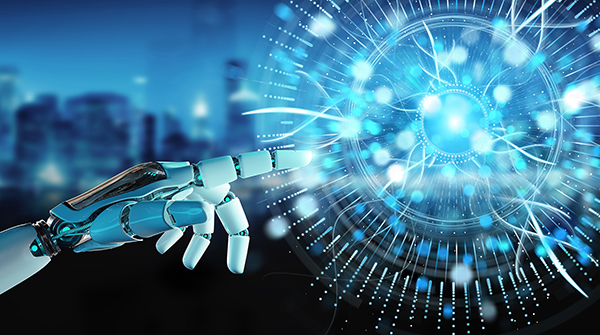
- 下一篇
- 流浪地球2:无人驾驶技术给图恒宇带来的不是灾难,而是无限趋近完美的探索
-
- 科技周边 · 人工智能 | 3小时前 | golang
- Golang对接Hugging Face模型 教你快速部署AI文本分类
- 437浏览 收藏
-
- 科技周边 · 人工智能 | 3小时前 |
- 官方认证指南!DeepSeek满血版免费使用入口与高阶功能详解
- 357浏览 收藏
-
- 科技周边 · 人工智能 | 3小时前 |
- ChatGPT能画图吗 图像生成功能使用指南
- 425浏览 收藏
-
- 科技周边 · 人工智能 | 3小时前 |
- Memo AI 网页端使用方法
- 179浏览 收藏
-
- 科技周边 · 人工智能 | 3小时前 |
- 怎么用豆包AI帮我写PHP Laravel路由 用AI构建RESTful API的秘诀
- 444浏览 收藏
-
- 科技周边 · 人工智能 | 3小时前 |
- 豆包AI反应慢怎么办 优化使用体验的实用建议
- 283浏览 收藏
-
- 科技周边 · 人工智能 | 3小时前 |
- 如何接入ChatGPT API ChatGPT API接入与调用教程
- 166浏览 收藏
-
- 科技周边 · 人工智能 | 3小时前 |
- 即梦ai如何添加时间戳 即梦ai日期水印设置指南
- 369浏览 收藏
-
- 科技周边 · 人工智能 | 3小时前 |
- Midjourney + Photoshop 精修教程:让人物AI图片更完美
- 497浏览 收藏
-
- 科技周边 · 人工智能 | 3小时前 |
- 如何使用Hugging Face的Transformer库 Hugging Face模型加载与推理教程
- 332浏览 收藏
-
- 前端进阶之JavaScript设计模式
- 设计模式是开发人员在软件开发过程中面临一般问题时的解决方案,代表了最佳的实践。本课程的主打内容包括JS常见设计模式以及具体应用场景,打造一站式知识长龙服务,适合有JS基础的同学学习。
- 542次学习
-
- GO语言核心编程课程
- 本课程采用真实案例,全面具体可落地,从理论到实践,一步一步将GO核心编程技术、编程思想、底层实现融会贯通,使学习者贴近时代脉搏,做IT互联网时代的弄潮儿。
- 508次学习
-
- 简单聊聊mysql8与网络通信
- 如有问题加微信:Le-studyg;在课程中,我们将首先介绍MySQL8的新特性,包括性能优化、安全增强、新数据类型等,帮助学生快速熟悉MySQL8的最新功能。接着,我们将深入解析MySQL的网络通信机制,包括协议、连接管理、数据传输等,让
- 497次学习
-
- JavaScript正则表达式基础与实战
- 在任何一门编程语言中,正则表达式,都是一项重要的知识,它提供了高效的字符串匹配与捕获机制,可以极大的简化程序设计。
- 487次学习
-
- 从零制作响应式网站—Grid布局
- 本系列教程将展示从零制作一个假想的网络科技公司官网,分为导航,轮播,关于我们,成功案例,服务流程,团队介绍,数据部分,公司动态,底部信息等内容区块。网站整体采用CSSGrid布局,支持响应式,有流畅过渡和展现动画。
- 484次学习
-
- 免费AI认证证书
- 科大讯飞AI大学堂推出免费大模型工程师认证,助力您掌握AI技能,提升职场竞争力。体系化学习,实战项目,权威认证,助您成为企业级大模型应用人才。
- 18次使用
-
- 茅茅虫AIGC检测
- 茅茅虫AIGC检测,湖南茅茅虫科技有限公司倾力打造,运用NLP技术精准识别AI生成文本,提供论文、专著等学术文本的AIGC检测服务。支持多种格式,生成可视化报告,保障您的学术诚信和内容质量。
- 160次使用
-
- 赛林匹克平台(Challympics)
- 探索赛林匹克平台Challympics,一个聚焦人工智能、算力算法、量子计算等前沿技术的赛事聚合平台。连接产学研用,助力科技创新与产业升级。
- 196次使用
-
- 笔格AIPPT
- SEO 笔格AIPPT是135编辑器推出的AI智能PPT制作平台,依托DeepSeek大模型,实现智能大纲生成、一键PPT生成、AI文字优化、图像生成等功能。免费试用,提升PPT制作效率,适用于商务演示、教育培训等多种场景。
- 177次使用
-
- 稿定PPT
- 告别PPT制作难题!稿定PPT提供海量模板、AI智能生成、在线协作,助您轻松制作专业演示文稿。职场办公、教育学习、企业服务全覆盖,降本增效,释放创意!
- 167次使用
-
- GPT-4王者加冕!读图做题性能炸天,凭自己就能考上斯坦福
- 2023-04-25 501浏览
-
- 单块V100训练模型提速72倍!尤洋团队新成果获AAAI 2023杰出论文奖
- 2023-04-24 501浏览
-
- ChatGPT 真的会接管世界吗?
- 2023-04-13 501浏览
-
- VR的终极形态是「假眼」?Neuralink前联合创始人掏出新产品:科学之眼!
- 2023-04-30 501浏览
-
- 实现实时制造可视性优势有哪些?
- 2023-04-15 501浏览