PyTorch 中的 CocoDetection (1)
来源:dev.to
2025-01-20 15:46:45
0浏览
收藏
本篇文章给大家分享《PyTorch 中的 CocoDetection (1)》,覆盖了文章的常见基础知识,其实一个语言的全部知识点一篇文章是不可能说完的,但希望通过这些问题,让读者对自己的掌握程度有一定的认识(B 数),从而弥补自己的不足,更好的掌握它。
请我喝杯咖啡☕
*备忘录:
- 我的帖子解释了cocodetection()使用train2017与captions_train2017.json,instances_train2017.json和person_keypoints_train2017.json,val2017与captions_val2017.json,instances_val2017.json和person_keypoints_val2017.json和test2017与image_info_test2017.json和image_info_test-dev2017.json.
- 我的帖子解释了 ms coco。
cocodetection() 可以使用 ms coco 数据集,如下所示。 *这适用于带有captions_train2014.json、instances_train2014.json和person_keypoints_train2014.json的train2014,带有captions_val2014.json、instances_val2014.json和person_keypoints_val2014.json的val2014以及带有image_info_test2014.json、image_info_test2015.json和的test2017 image_info_test-dev2015.json:
*备忘录:
- 第一个参数是root(必需类型:str或pathlib.path):
*备注:
- 这是图像的路径。
- 绝对或相对路径都是可能的。
- 第二个参数是 annfile(必需类型:str 或 pathlib.path):
*备注:
- 这是注释的路径。
- 绝对或相对路径都是可能的。
- 第三个参数是transform(optional-default:none-type:callable)。
- 第四个参数是 target_transform(optional-default:none-type:callable)。
- 第五个参数是transforms(optional-default:none-type:callable)。
from torchvision.datasets import CocoDetection cap_train2014_data = CocoDetection( root="data/coco/imgs/train2014", annFile="data/coco/anns/trainval2014/captions_train2014.json" ) cap_train2014_data = CocoDetection( root="data/coco/imgs/train2014", annFile="data/coco/anns/trainval2014/captions_train2014.json", transform=None, target_transform=None, transforms=None ) ins_train2014_data = CocoDetection( root="data/coco/imgs/train2014", annFile="data/coco/anns/trainval2014/instances_train2014.json" ) pk_train2014_data = CocoDetection( root="data/coco/imgs/train2014", annFile="data/coco/anns/trainval2014/person_keypoints_train2014.json" ) len(cap_train2014_data), len(ins_train2014_data), len(pk_train2014_data) # (82783, 82783, 82783) cap_val2014_data = CocoDetection( root="data/coco/imgs/val2014", annFile="data/coco/anns/trainval2014/captions_val2014.json" ) ins_val2014_data = CocoDetection( root="data/coco/imgs/val2014", annFile="data/coco/anns/trainval2014/instances_val2014.json" ) pk_val2014_data = CocoDetection( root="data/coco/imgs/val2014", annFile="data/coco/anns/trainval2014/person_keypoints_val2014.json" ) len(cap_val2014_data), len(ins_val2014_data), len(pk_val2014_data) # (40504, 40504, 40504) test2014_data = CocoDetection( root="data/coco/imgs/test2014", annFile="data/coco/anns/test2014/image_info_test2014.json" ) test2015_data = CocoDetection( root="data/coco/imgs/test2015", annFile="data/coco/anns/test2015/image_info_test2015.json" ) testdev2015_data = CocoDetection( root="data/coco/imgs/test2015", annFile="data/coco/anns/test2015/image_info_test-dev2015.json" ) len(test2014_data), len(test2015_data), len(testdev2015_data) # (40775, 81434, 20288) cap_train2014_data # Dataset CocoDetection # Number of datapoints: 82783 # Root location: data/coco/imgs/train2014 cap_train2014_data.root # 'data/coco/imgs/train2014' print(cap_train2014_data.transform) # None print(cap_train2014_data.target_transform) # None print(cap_train2014_data.transforms) # None cap_train2014_data.coco # <pycocotools.coco.COCO at 0x7c8a5f09d4f0> cap_train2014_data[26] # (<PIL.Image.Image image mode=RGB size=427x640>, # [{'image_id': 154, 'id': 202466, # 'caption': 'three zeebras standing in a grassy field walking'}, # {'image_id': 154, 'id': 211904, # 'caption': 'Three zebras are standing in an open field.'}, # {'image_id': 154, 'id': 215654, # 'caption': 'Three zebra are walking through the grass of a field.'}, # {'image_id': 154, 'id': 216620, # 'caption': 'Three zebras standing on a grassy dirt field.'}, # {'image_id': 154, 'id': 231686, # 'caption': 'Three zebras grazing in green grass field area.'}]) cap_train2014_data[179] # (<PIL.Image.Image image mode=RGB size=480x640>, # [{'image_id': 1330, 'id': 721877, # 'caption': 'a young guy walking in a forrest holding ... his hand'}, # {'image_id': 1330, 'id': 727442, # 'caption': 'A partially black and white photo of a ... the woods.'}, # {'image_id': 1330, 'id': 730133, # 'caption': 'A disc golfer releases a throw ... wooded course.'}, # {'image_id': 1330, 'id': 731450, # 'caption': 'The person is in the clearing of a wooded area. '}, # {'image_id': 1330, 'id': 732335, # 'caption': 'a person throwing a frisbee at many trees '}]) cap_train2014_data[194] # (<PIL.Image.Image image mode=RGB size=428x640>, # [{'image_id': 1407, 'id': 451510, # 'caption': 'A person on a court with a tennis racket.'}, # {'image_id': 1407, 'id': 457735, # 'caption': 'A man that is holding a racquet ... the grass.'}, # {'image_id': 1407, 'id': 460600, # 'caption': 'A tennis player hits the ball during a match.'}, # {'image_id': 1407, 'id': 460612, # 'caption': 'The tennis player is poised to serve a ball.'}, # {'image_id': 1407, 'id': 821947, # 'caption': 'Man in white playing tennis on a court.'}]) ins_train2014_data[26] # (<PIL.Image.Image image mode=RGB size=427x640>, # [{'segmentation': [[229.5, 618.18, 235.64, ..., 219.85, 618.18]], # 'area': 53702.50415, 'iscrowd': 0, 'image_id': 154, # 'bbox': [11.98, 315.59, 349.08, 324.41], 'category_id': 24, # 'id': 590410}, # {'segmentation': ..., 'category_id': 24, 'id': 590623}, # {'segmentation': ..., 'category_id': 24, 'id': 593205}]) ins_train2014_data[179] # (<PIL.Image.Image image mode=RGB size=480x640>, # [{'segmentation': [[160.87, 574.0, 174.15, ..., 162.77, 577.6]], # 'area': 21922.32225, 'iscrowd': 0, 'image_id': 1330, # 'bbox': [38.47, 228.02, 249.55, 349.58], 'category_id': 1, # 'id': 497247}, # {'segmentation': ..., 'category_id': 34, 'id': 604179}]) ins_train2014_data[194] # (<PIL.Image.Image image mode=RGB size=428x640>, # [{'segmentation': [[203.26, 465.95, 215.13, ..., 207.22, 466.94]], # 'area': 20449.62315, 'iscrowd': 0, 'image_id': 1407, # 'bbox': [138.97, 198.88, 175.08, 355.11], 'category_id': 1, # 'id': 434962}, # {'segmentation': ..., 'category_id': 43, 'id': 658155}, # ... # {'segmentation': ..., 'category_id': 1, 'id': 2000535}]) pk_train2014_data[26] # (<PIL.Image.Image image mode=RGB size=427x640>, []) pk_train2014_data[179] # (<PIL.Image.Image image mode=RGB size=480x640>, # [{'segmentation': [[160.87, 574, 174.15, ..., 162.77, 577.6]], # 'num_keypoints': 14, 'area': 21922.32225, 'iscrowd': 0, # 'keypoints': [0, 0, 0, 0, ..., 510, 2], 'image_id': 1330, # 'bbox': [38.47, 228.02, 249.55, 349.58], 'category_id': 1, # 'id': 497247}]) pk_train2014_data[194] # (<PIL.Image.Image image mode=RGB size=428x640>, # [{'segmentation': [[203.26, 465.95, 215.13, ..., 207.22, 466.94]], # 'num_keypoints': 16, 'area': 20449.62315, 'iscrowd': 0, # 'keypoints': [243, 289, 2, 247, ..., 516, 2], 'image_id': 1407, # 'bbox': [138.97, 198.88, 175.08, 355.11], 'category_id': 1, # 'id': 434962}, # {'segmentation': ..., 'category_id': 1, 'id': 1246131}, # ... # {'segmentation': ..., 'category_id': 1, 'id': 2000535}]) cap_val2014_data[26] # (<PIL.Image.Image image mode=RGB size=640x360>, # [{'image_id': 428, 'id': 281051, # 'caption': 'a close up of a child next to a cake with balloons'}, # {'image_id': 428, 'id': 283808, # 'caption': 'A baby sitting in front of a cake wearing a tie.'}, # {'image_id': 428, 'id': 284135, # 'caption': 'The young boy is dressed in a tie that ... his cake. '}, # {'image_id': 428, 'id': 284627, # 'caption': 'A child eating a birthday cake near some balloons.'}, # {'image_id': 428, 'id': 401924, # 'caption': 'A baby eating a cake with a tie ... the background.'}]) cap_val2014_data[179] # (<PIL.Image.Image image mode=RGB size=500x302>, # [{'image_id': 2299, 'id': 692974, # 'caption': 'Many small children are posing ... white photo. '}, # {'image_id': 2299, 'id': 693640, # 'caption': 'A vintage school picture of grade school aged children.'}, # {'image_id': 2299, 'id': 694699, # 'caption': 'A black and white photo of a group of kids.'}, # {'image_id': 2299, 'id': 697432, # 'caption': 'A group of children standing next to each other.'}, # {'image_id': 2299, 'id': 698791, # 'caption': 'A group of children standing and ... each other. '}]) cap_val2014_data[194] # (<PIL.Image.Image image mode=RGB size=640x427>, # [{'image_id': 2562, 'id': 267259, # 'caption': 'A man hitting a tennis ball with a racquet.'}, # {'image_id': 2562, 'id': 277075, # 'caption': 'champion tennis player swats at the ball ... to win'}, # {'image_id': 2562, 'id': 279091, # 'caption': 'A man is hitting his tennis ball with ... the court.'}, # {'image_id': 2562, 'id': 406135, # 'caption': 'a tennis player on a court with a racket'}, # {'image_id': 2562, 'id': 823086, # 'caption': 'A professional tennis player hits a ... fans watch.'}]) ins_val2014_data[26] # (<PIL.Image.Image image mode=RGB size=640x360>, # [{'segmentation': [[378.61, 210.2, 409.35, ..., 374.56, 217.48]], # 'area': 3573.3858000000005, 'iscrowd': 0, 'image_id': 428, # 'bbox': [374.56, 200.49, 94.65, 154.52], 'category_id': 32, # 'id': 293908}, # {'segmentation': ..., 'category_id': 1, 'id': 487626}, # {'segmentation': ..., 'category_id': 61, 'id': 1085469}]) ins_val2014_data[179] # (<PIL.Image.Image image mode=RGB size=500x302>, # [{'segmentation': [[107.49, 226.51, 108.17, ..., 105.8, 226.43]], # 'area': 66.15510000000003, 'iscrowd': 0, 'image_id': 2299, # 'bbox': [101.74, 226.43, 7.53, 15.83], 'category_id': 32, # 'id': 295960}, # {'segmentation': ..., 'category_id': 32, 'id': 298359}, # ... # {'segmentation': {'counts': [152, 13, 263, 40, 2, ..., 132, 75], # 'size': [302, 500]}, 'area': 87090, 'iscrowd': 1, 'image_id': 2299, # 'bbox': [0, 18, 499, 263], 'category_id': 1, 'id': 900100002299}]) ins_val2014_data[194] # (<PIL.Image.Image image mode=RGB size=640x427>, # [{'segmentation': [[389.92, 6.17, 391.48, ..., 393.57, 0.57]], # 'area': 482.5815999999996, 'iscrowd': 0, 'image_id': 2562, # 'bbox': [389.92, 0.57, 28.15, 21.38], 'category_id': 37, # 'id': 302161}, # {'segmentation': ..., 'category_id': 43, 'id': 659770}, # ... # {'segmentation': {'counts': [132, 8, 370, 37, 3, ..., 82, 268], # 'size': [427, 640]}, 'area': 19849, 'iscrowd': 1, 'image_id': 2562, # 'bbox': [0, 49, 639, 193], 'category_id': 1, 'id': 900100002562}]) pk_val2014_data[26] # (<PIL.Image.Image image mode=RGB size=640x360>, # [{'segmentation': [[239.18, 244.08, 229.39, ..., 256.33, 251.43]], # 'num_keypoints': 10, 'area': 55007.0814, 'iscrowd': 0, # 'keypoints': [383, 132, 2, 418, ..., 0, 0], 'image_id': 428, # 'bbox': [226.94, 32.65, 355.92, 323.27], 'category_id': 1, # 'id': 487626}]) pk_val2014_data[179] # (<PIL.Image.Image image mode=RGB size=500x302>, # [{'segmentation': [[75, 272.02, 76.92, ..., 74.67, 272.66]], # 'num_keypoints': 17, 'area': 4357.5248, 'iscrowd': 0, # 'keypoints': [108, 213, 2, 113, ..., 289, 2], 'image_id': 2299, # 'bbox': [70.18, 189.51, 64.2, 112.04], 'category_id': 1, # 'id': 1219726}, # {'segmentation': ..., 'category_id': 1, 'id': 1226789}, # ... # {'segmentation': {'counts': [152, 13, 263, 40, 2, ..., 132, 75], # 'size': [302, 500]}, 'num_keypoints': 0, 'area': 87090, # 'iscrowd': 1, 'keypoints': [0, 0, 0, 0, ..., 0, 0], 'image_id': 2299, # 'bbox': [0, 18, 499, 263], 'category_id': 1, 'id': 900100002299}]) pk_val2014_data[194] # (<PIL.Image.Image image mode=RGB size=640x427>, # [{'segmentation': [[19.26, 270.62, 4.3, ..., 25.98, 273.61]], # 'num_keypoints': 13, 'area': 6008.95835, 'iscrowd': 0, # 'keypoints': [60, 160, 2, 64, ..., 257, 1], 'image_id': 2562, # 'bbox': [4.3, 144.26, 100.19, 129.35], 'category_id': 1, # 'id': 1287168}, # {'segmentation': ..., 'category_id': 1, 'id': 1294190}, # ... # {'segmentation': {'counts': [132, 8, 370, 37, 3, ..., 82, 268], # 'size': [427, 640]}, 'num_keypoints': 0, 'area': 19849, 'iscrowd': 1, # 'keypoints': [0, 0, 0, 0, ..., 0, 0], 'image_id': 2562, # 'bbox': [0, 49, 639, 193], 'category_id': 1, 'id': 900100002562}]) test2014_data[26] # (<PIL.Image.Image image mode=RGB size=640x640>, []) test2014_data[179] # (<PIL.Image.Image image mode=RGB size=640x480>, []) test2014_data[194] # (<PIL.Image.Image image mode=RGB size=640x360>, []) test2015_data[26] # (<PIL.Image.Image image mode=RGB size=640x480>, []) test2015_data[179] # (<PIL.Image.Image image mode=RGB size=640x426>, []) test2015_data[194] # (<PIL.Image.Image image mode=RGB size=640x480>, []) testdev2015_data[26] # (<PIL.Image.Image image mode=RGB size=640x360>, []) testdev2015_data[179] # (<PIL.Image.Image image mode=RGB size=640x480>, []) testdev2015_data[194] # (<PIL.Image.Image image mode=RGB size=640x480>, []) import matplotlib.pyplot as plt from matplotlib.patches import Polygon, Rectangle import numpy as np from pycocotools import mask # `show_images1()` doesn't work very well for the images with # segmentations and keypoints so for them, use `show_images2()` which # more uses the original coco functions. def show_images1(data, ims, main_title=None): file = data.root.split('/')[-1] fig, axes = plt.subplots(nrows=1, ncols=3, figsize=(14, 8)) fig.suptitle(t=main_title, y=0.9, fontsize=14) x_crd = 0.02 for i, axis in zip(ims, axes.ravel()): if data[i][1] and "caption" in data[i][1][0]: im, anns = data[i] axis.imshow(X=im) axis.set_title(label=anns[0]["image_id"]) y_crd = 0.0 for ann in anns: text_list = ann["caption"].split() if len(text_list) > 9: text = " ".join(text_list[0:10]) + " ..." else: text = " ".join(text_list) plt.figtext(x=x_crd, y=y_crd, fontsize=10, s=f'{ann["id"]}:\n{text}') y_crd -= 0.06 x_crd += 0.325 if i == 2 and file == "val2017": x_crd += 0.06 if data[i][1] and "segmentation" in data[i][1][0]: im, anns = data[i] axis.imshow(X=im) axis.set_title(label=anns[0]["image_id"]) for ann in anns: if "counts" in ann['segmentation']: seg = ann['segmentation'] # rle is Run Length Encoding. uncompressed_rle = [seg['counts']] height, width = seg['size'] compressed_rle = mask.frPyObjects(pyobj=uncompressed_rle, h=height, w=width) # rld is Run Length Decoding. compressed_rld = mask.decode(rleObjs=compressed_rle) y_plts, x_plts = np.nonzero(a=np.squeeze(a=compressed_rld)) axis.plot(x_plts, y_plts, color='yellow') else: for seg in ann['segmentation']: seg_arrs = np.split(ary=np.array(seg), indices_or_sections=len(seg)/2) poly = Polygon(xy=seg_arrs, facecolor="lightgreen", alpha=0.7) axis.add_patch(p=poly) x_plts = [seg_arr[0] for seg_arr in seg_arrs] y_plts = [seg_arr[1] for seg_arr in seg_arrs] axis.plot(x_plts, y_plts, color='yellow') x, y, w, h = ann['bbox'] rect = Rectangle(xy=(x, y), width=w, height=h, linewidth=3, edgecolor='r', facecolor='none', zorder=2) axis.add_patch(p=rect) if data[i][1] and 'keypoints' in data[i][1][0]: kps = ann['keypoints'] kps_arrs = np.split(ary=np.array(kps), indices_or_sections=len(kps)/3) x_plts = [kps_arr[0] for kps_arr in kps_arrs] y_plts = [kps_arr[1] for kps_arr in kps_arrs] nonzeros_x_plts = [] nonzeros_y_plts = [] for x_plt, y_plt in zip(x_plts, y_plts): if x_plt == 0 and y_plt == 0: continue nonzeros_x_plts.append(x_plt) nonzeros_y_plts.append(y_plt) axis.scatter(x=nonzeros_x_plts, y=nonzeros_y_plts, color='yellow') # ↓ ↓ ↓ ↓ ↓ ↓ ↓ ↓ Bad result ↓ ↓ ↓ ↓ ↓ ↓ ↓ ↓ # axis.plot(nonzeros_x_plts, nonzeros_y_plts) if not data[i][1]: im, _ = data[i] axis.imshow(X=im) fig.tight_layout() plt.show() ims = (26, 179, 194) show_images1(data=cap_train2014_data, ims=ims, main_title="cap_train2014_data") show_images1(data=ins_train2014_data, ims=ims, main_title="ins_train2014_data") show_images1(data=pk_train2014_data, ims=ims, main_title="pk_train2014_data") print() show_images1(data=cap_val2014_data, ims=ims, main_title="cap_val2014_data") show_images1(data=ins_val2014_data, ims=ims, main_title="ins_val2014_data") show_images1(data=pk_val2014_data, ims=ims, main_title="pk_val2014_data") print() show_images1(data=test2014_data, ims=ims, main_title="test2014_data") show_images1(data=test2015_data, ims=ims, main_title="test2015_data") show_images1(data=testdev2015_data, ims=ims, main_title="testdev2015_data") # `show_images2()` works very well for the images with segmentations and # keypoints. def show_images2(data, index, main_title=None): img_set = data[index] img, img_anns = img_set if img_anns and "segmentation" in img_anns[0]: img_id = img_anns[0]['image_id'] coco = data.coco def show_image(imgIds, areaRng=[], iscrowd=None, draw_bbox=False): plt.figure(figsize=(11, 6)) plt.imshow(X=img) plt.suptitle(t=main_title, y=1, fontsize=14) plt.title(label=img_id, fontsize=14) anns_ids = coco.getAnnIds(imgIds=img_id, areaRng=areaRng, iscrowd=iscrowd) anns = coco.loadAnns(ids=anns_ids) coco.showAnns(anns=anns, draw_bbox=draw_bbox) plt.show() show_image(imgIds=img_id, draw_bbox=True) show_image(imgIds=img_id, draw_bbox=False) show_image(imgIds=img_id, iscrowd=False, draw_bbox=True) show_image(imgIds=img_id, areaRng=[0, 5000], draw_bbox=True) elif img_anns and not "segmentation" in img_anns[0]: plt.figure(figsize=(11, 6)) img_id = img_anns[0]['image_id'] plt.imshow(X=img) plt.suptitle(t=main_title, y=1, fontsize=14) plt.title(label=img_id, fontsize=14) plt.show() elif not img_anns: plt.figure(figsize=(11, 6)) plt.imshow(X=img) plt.suptitle(t=main_title, y=1, fontsize=14) plt.show() show_images2(data=ins_val2014_data, index=179, main_title="ins_val2014_data") print() show_images2(data=pk_val2014_data, index=179, main_title="pk_val2014_data") print() show_images2(data=ins_val2014_data, index=194, main_title="ins_val2014_data") print() show_images2(data=pk_val2014_data, index=194, main_title="pk_val2014_data")
显示_图像1():
显示图像2():
文中关于的知识介绍,希望对你的学习有所帮助!若是受益匪浅,那就动动鼠标收藏这篇《PyTorch 中的 CocoDetection (1)》文章吧,也可关注golang学习网公众号了解相关技术文章。
版本声明
本文转载于:dev.to 如有侵犯,请联系study_golang@163.com删除
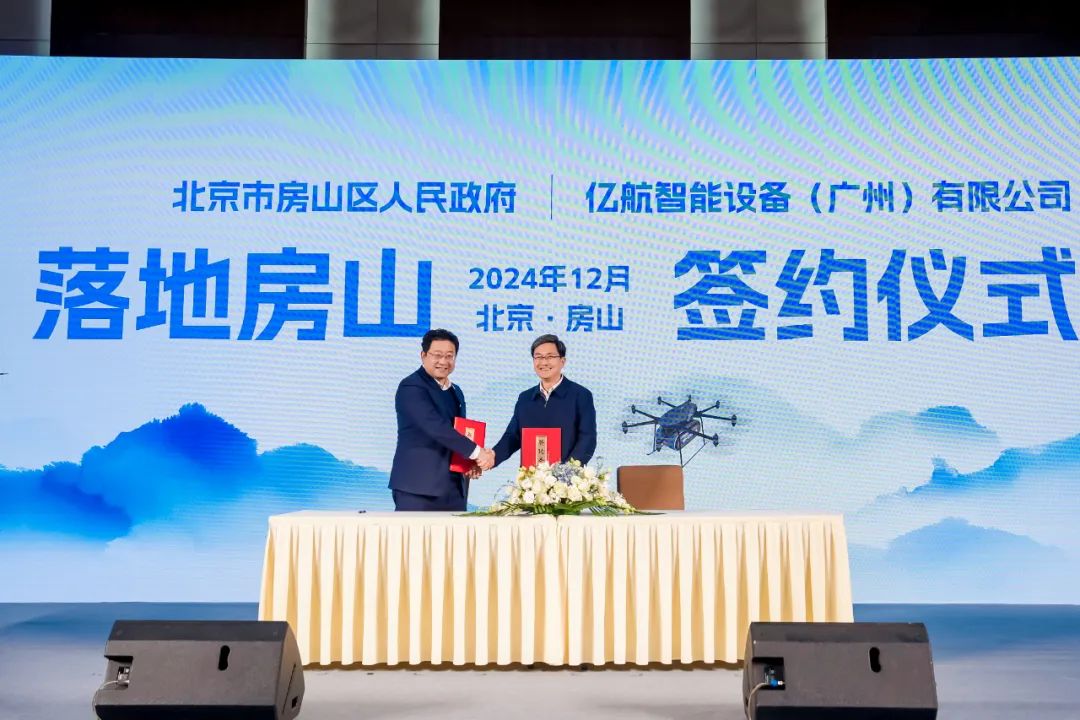
- 上一篇
- 亿航智能将在北京设立低空应急救援装备全国总部
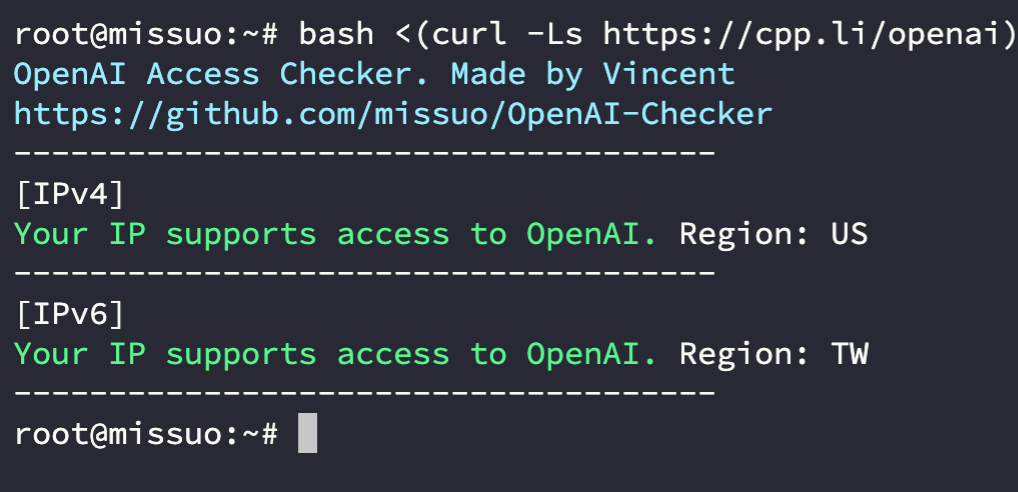
- 下一篇
- 检测使用的IP是否支持使用 ChatGPT
查看更多
最新文章
-
- 文章 · python教程 | 5小时前 |
- Python正则匹配Unicode字符技巧
- 346浏览 收藏
-
- 文章 · python教程 | 5小时前 |
- Python中lambda的用法及匿名函数创建方法
- 127浏览 收藏
-
- 文章 · python教程 | 5小时前 |
- PyCharm运行代码方法详解
- 372浏览 收藏
-
- 文章 · python教程 | 6小时前 |
- Python中eval的作用与使用详解
- 244浏览 收藏
-
- 文章 · python教程 | 6小时前 |
- 正则表达式量词有哪些及用法详解
- 391浏览 收藏
-
- 文章 · python教程 | 6小时前 | Python 链式比较
- Python链式比较技巧,简化条件判断方法
- 225浏览 收藏
-
- 文章 · python教程 | 7小时前 |
- PyCharm解释器选择指南与建议
- 174浏览 收藏
-
- 文章 · python教程 | 7小时前 |
- 模块导入错误:ImportError与ModuleNotFoundError区别详解
- 339浏览 收藏
-
- 文章 · python教程 | 7小时前 |
- PyCharm中文界面设置教程3步搞定
- 317浏览 收藏
-
- 文章 · python教程 | 7小时前 |
- Python分页实现与优化技巧分享
- 384浏览 收藏
-
- 文章 · python教程 | 7小时前 |
- Pythonunittest使用教程详解
- 139浏览 收藏
-
- 文章 · python教程 | 7小时前 |
- Python类型注解教程与实用技巧
- 463浏览 收藏
查看更多
课程推荐
-
- 前端进阶之JavaScript设计模式
- 设计模式是开发人员在软件开发过程中面临一般问题时的解决方案,代表了最佳的实践。本课程的主打内容包括JS常见设计模式以及具体应用场景,打造一站式知识长龙服务,适合有JS基础的同学学习。
- 542次学习
-
- GO语言核心编程课程
- 本课程采用真实案例,全面具体可落地,从理论到实践,一步一步将GO核心编程技术、编程思想、底层实现融会贯通,使学习者贴近时代脉搏,做IT互联网时代的弄潮儿。
- 508次学习
-
- 简单聊聊mysql8与网络通信
- 如有问题加微信:Le-studyg;在课程中,我们将首先介绍MySQL8的新特性,包括性能优化、安全增强、新数据类型等,帮助学生快速熟悉MySQL8的最新功能。接着,我们将深入解析MySQL的网络通信机制,包括协议、连接管理、数据传输等,让
- 497次学习
-
- JavaScript正则表达式基础与实战
- 在任何一门编程语言中,正则表达式,都是一项重要的知识,它提供了高效的字符串匹配与捕获机制,可以极大的简化程序设计。
- 487次学习
-
- 从零制作响应式网站—Grid布局
- 本系列教程将展示从零制作一个假想的网络科技公司官网,分为导航,轮播,关于我们,成功案例,服务流程,团队介绍,数据部分,公司动态,底部信息等内容区块。网站整体采用CSSGrid布局,支持响应式,有流畅过渡和展现动画。
- 484次学习
查看更多
AI推荐
-
- 免费AI认证证书
- 科大讯飞AI大学堂推出免费大模型工程师认证,助力您掌握AI技能,提升职场竞争力。体系化学习,实战项目,权威认证,助您成为企业级大模型应用人才。
- 12次使用
-
- 茅茅虫AIGC检测
- 茅茅虫AIGC检测,湖南茅茅虫科技有限公司倾力打造,运用NLP技术精准识别AI生成文本,提供论文、专著等学术文本的AIGC检测服务。支持多种格式,生成可视化报告,保障您的学术诚信和内容质量。
- 157次使用
-
- 赛林匹克平台(Challympics)
- 探索赛林匹克平台Challympics,一个聚焦人工智能、算力算法、量子计算等前沿技术的赛事聚合平台。连接产学研用,助力科技创新与产业升级。
- 187次使用
-
- 笔格AIPPT
- SEO 笔格AIPPT是135编辑器推出的AI智能PPT制作平台,依托DeepSeek大模型,实现智能大纲生成、一键PPT生成、AI文字优化、图像生成等功能。免费试用,提升PPT制作效率,适用于商务演示、教育培训等多种场景。
- 174次使用
-
- 稿定PPT
- 告别PPT制作难题!稿定PPT提供海量模板、AI智能生成、在线协作,助您轻松制作专业演示文稿。职场办公、教育学习、企业服务全覆盖,降本增效,释放创意!
- 161次使用
查看更多
相关文章
-
- Flask框架安装技巧:让你的开发更高效
- 2024-01-03 501浏览
-
- Django框架中的并发处理技巧
- 2024-01-22 501浏览
-
- 提升Python包下载速度的方法——正确配置pip的国内源
- 2024-01-17 501浏览
-
- Python与C++:哪个编程语言更适合初学者?
- 2024-03-25 501浏览
-
- 品牌建设技巧
- 2024-04-06 501浏览