PyTorch 中的子项目
来源:dev.to
2025-01-14 19:33:12
0浏览
收藏
各位小伙伴们,大家好呀!看看今天我又给各位带来了什么文章?本文标题是《PyTorch 中的子项目》,很明显是关于文章的文章哈哈哈,其中内容主要会涉及到等等,如果能帮到你,觉得很不错的话,欢迎各位多多点评和分享!
请我喝杯咖啡☕
*备忘录:
- 我的帖子解释了 add()。
- 我的帖子解释了 mul()。
- 我的帖子解释了 div()。
- 我的帖子解释了余数()。
- 我的帖子解释了 fmod()。
sub() 可以与零个或多个元素或标量的 0d 或多个 d 张量中的两个或零个或多个元素的 0d 或多个 d 张量与一个标量进行减法,得到为零的 0d 或多个 d 张量或更多元素,如下所示:
*备忘录:
- sub() 可以与 torch 或张量一起使用。
- 第一个参数(输入)带有 torch(类型:int、float 或complex 的张量或标量)或使用张量(类型:int、float 或complex 的张量)(必需)。
- 带有 torch 的第二个参数或带有张量的第一个参数是其他(必需类型:张量或 int、float 或complex 标量)。
- 带有 torch 的第三个参数或带有张量的第二个参数是 alpha(可选-默认:1-类型:张量或整数、浮点或复数标量)。 *other 乘以 alpha(输入或张量 -(otherxalpha))。
- torch 存在 out 参数(可选-默认:无-类型:张量):
*备注:
- 必须使用 out=。
- 我的帖子解释了论点。
- minus() 是 sub() 的别名。
import torch tensor1 = torch.tensor([9, 7, 6]) tensor2 = torch.tensor([[4, -4, 3], [-2, 5, -5]]) torch.sub(input=tensor1, other=tensor2) tensor1.sub(other=tensor2) torch.sub(input=tensor1, other=tensor2, alpha=1) torch.sub(input=tensor1, other=tensor2, alpha=torch.tensor(1)) # tensor([[5, 11, 3], [11, 2, 11]]) torch.sub(input=tensor1, other=tensor2, alpha=0) torch.sub(input=tensor1, other=tensor2, alpha=torch.tensor(0)) # tensor([[9, 7, 6], [9, 7, 6]]) torch.sub(input=tensor1, other=tensor2, alpha=2) torch.sub(input=tensor1, other=tensor2, alpha=torch.tensor(2)) # tensor([[1, 15, 0], [13, -3, 16]]) torch.sub(input=tensor1, other=tensor2, alpha=-1) torch.sub(input=tensor1, other=tensor2, alpha=torch.tensor(-1)) # tensor([[13, 3, 9], [7, 12, 1]]) torch.sub(input=tensor1, other=tensor2, alpha=-2) torch.sub(input=tensor1, other=tensor2, alpha=torch.tensor(-2)) # tensor([[17, -1, 12], [5, 17, -4]]) torch.sub(input=9, other=tensor2) torch.sub(input=9, other=tensor2, alpha=1) torch.sub(input=9, other=tensor2, alpha=torch.tensor(1)) # tensor([[5, 13, 6], [11, 4, 14]]) torch.sub(input=tensor1, other=4) torch.sub(input=tensor1, other=4, alpha=1) torch.sub(input=tensor1, other=4, alpha=torch.tensor(1)) # tensor([5, 3, 2]) torch.sub(input=9, other=4) torch.sub(input=9, other=4, alpha=1) torch.sub(input=9, other=4, alpha=torch.tensor(1)) # tensor(5) tensor1 = torch.tensor([9., 7., 6.]) tensor2 = torch.tensor([[4., -4., 3.], [-2., 5., -5.]]) torch.sub(input=tensor1, other=tensor2) torch.sub(input=tensor1, other=tensor2, alpha=1.) torch.sub(input=tensor1, other=tensor2, alpha=torch.tensor(1.)) # tensor([[5., 11., 3.], [11., 2., 11.]]) torch.sub(input=9., other=tensor2) torch.sub(input=9., other=tensor2, alpha=1.) torch.sub(input=9., other=tensor2, alpha=torch.tensor(1.)) # tensor([[5., 13., 6.], [11., 4., 14.]]) torch.sub(input=tensor1, other=4) torch.sub(input=tensor1, other=4, alpha=1.) torch.sub(input=tensor1, other=4, alpha=torch.tensor(1.)) # tensor([5., 3., 2.]) torch.sub(input=9., other=4) torch.sub(input=9., other=4, alpha=1.) torch.sub(input=9., other=4, alpha=torch.tensor(1.)) # tensor(5.) tensor1 = torch.tensor([9.+0.j, 7.+0.j, 6.+0.j]) tensor2 = torch.tensor([[4.+0.j, -4.+0.j, 3.+0.j], [-2.+0.j, 5.+0.j, -5.+0.j]]) torch.sub(input=tensor1, other=tensor2) torch.sub(input=tensor1, other=tensor2, alpha=1.+0.j) torch.sub(input=tensor1, other=tensor2, alpha=torch.tensor(1.+0.j)) # tensor([[5.+0.j, 11.+0.j, 3.+0.j], # [11.+0.j, 2.+0.j, 11.+0.j]]) torch.sub(input=9.+0.j, other=tensor2) torch.sub(input=9.+0.j, other=tensor2, alpha=1.+0.j) torch.sub(input=9.+0.j, other=tensor2, alpha=torch.tensor(1.+0.j)) # tensor([[5.+0.j, 13.+0.j, 6.+0.j], # [11.+0.j, 4.+0.j, 14.+0.j]]) torch.sub(input=tensor1, other=4.+0.j) torch.sub(input=tensor1, other=4.+0.j, alpha=1.+0.j) torch.sub(input=tensor1, other=4.+0.j, alpha=torch.tensor(1.+0.j)) # tensor([5.+0.j, 3.+0.j, 2.+0.j]) torch.sub(input=9.+0.j, other=4.+0.j) torch.sub(input=9.+0.j, other=4.+0.j, alpha=1.+0.j) torch.sub(input=9.+0.j, other=4.+0.j, alpha=torch.tensor(1.+0.j)) # tensor(5.+0.j)
理论要掌握,实操不能落!以上关于《PyTorch 中的子项目》的详细介绍,大家都掌握了吧!如果想要继续提升自己的能力,那么就来关注golang学习网公众号吧!
版本声明
本文转载于:dev.to 如有侵犯,请联系study_golang@163.com删除
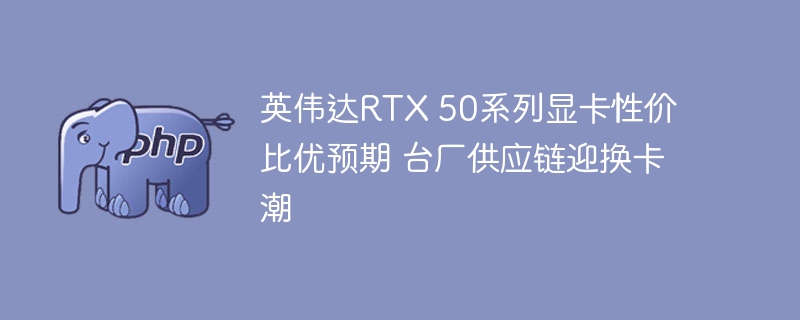
- 上一篇
- 英伟达RTX 50系列显卡性价比优预期 台厂供应链迎换卡潮
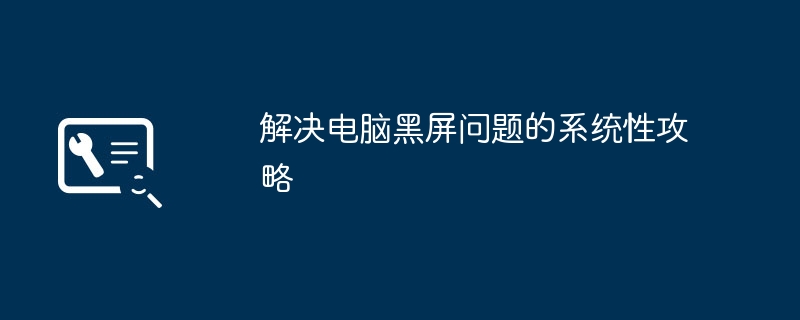
- 下一篇
- 解决电脑黑屏问题的系统性攻略
查看更多
最新文章
-
- 文章 · python教程 | 4小时前 | Excel文件 Pandas openpyxl read_excel chunksize
- Python处理Excel文件的实用技巧及方法
- 183浏览 收藏
-
- 文章 · python教程 | 5小时前 |
- 列表、元组、集合、字典遍历终极攻略
- 224浏览 收藏
-
- 文章 · python教程 | 5小时前 |
- FastAPI依赖注入的Python实用技巧
- 191浏览 收藏
-
- 文章 · python教程 | 5小时前 |
- Python模块导入与使用技巧大全
- 191浏览 收藏
-
- 文章 · python教程 | 5小时前 | JSON 数据处理 beautifulsoup Pandas xml.etree.ElementTree
- Python爬虫数据处理技巧及方法
- 459浏览 收藏
-
- 文章 · python教程 | 6小时前 |
- Python追加文件内容的简易技巧
- 205浏览 收藏
-
- 文章 · python教程 | 6小时前 | Numpy 切片 负索引 列表索引 IndexError
- Python数组索引的实现技巧
- 248浏览 收藏
-
- 文章 · python教程 | 6小时前 |
- 数据类型转换技巧及实战指南
- 359浏览 收藏
-
- 文章 · python教程 | 7小时前 | Matplotlib Seaborn Pandas scatterplot boxplot
- Pythonseaborn库使用方法与技巧大全
- 248浏览 收藏
-
- 文章 · python教程 | 7小时前 |
- 数据格式化输出技巧及攻略
- 196浏览 收藏
-
- 文章 · python教程 | 8小时前 | Python 可维护性 多态 鸭子类型 functools.singledispatch
- Python多态实现技巧与方法大全
- 464浏览 收藏
-
- 文章 · python教程 | 8小时前 | beautifulsoup 栈溢出 递归方法 迭代方法 DOM树遍历
- Python高效遍历DOM树的技巧
- 434浏览 收藏
查看更多
课程推荐
-
- 前端进阶之JavaScript设计模式
- 设计模式是开发人员在软件开发过程中面临一般问题时的解决方案,代表了最佳的实践。本课程的主打内容包括JS常见设计模式以及具体应用场景,打造一站式知识长龙服务,适合有JS基础的同学学习。
- 542次学习
-
- GO语言核心编程课程
- 本课程采用真实案例,全面具体可落地,从理论到实践,一步一步将GO核心编程技术、编程思想、底层实现融会贯通,使学习者贴近时代脉搏,做IT互联网时代的弄潮儿。
- 508次学习
-
- 简单聊聊mysql8与网络通信
- 如有问题加微信:Le-studyg;在课程中,我们将首先介绍MySQL8的新特性,包括性能优化、安全增强、新数据类型等,帮助学生快速熟悉MySQL8的最新功能。接着,我们将深入解析MySQL的网络通信机制,包括协议、连接管理、数据传输等,让
- 497次学习
-
- JavaScript正则表达式基础与实战
- 在任何一门编程语言中,正则表达式,都是一项重要的知识,它提供了高效的字符串匹配与捕获机制,可以极大的简化程序设计。
- 487次学习
-
- 从零制作响应式网站—Grid布局
- 本系列教程将展示从零制作一个假想的网络科技公司官网,分为导航,轮播,关于我们,成功案例,服务流程,团队介绍,数据部分,公司动态,底部信息等内容区块。网站整体采用CSSGrid布局,支持响应式,有流畅过渡和展现动画。
- 484次学习
查看更多
AI推荐
-
- 笔灵AI生成答辩PPT
- 探索笔灵AI生成答辩PPT的强大功能,快速制作高质量答辩PPT。精准内容提取、多样模板匹配、数据可视化、配套自述稿生成,让您的学术和职场展示更加专业与高效。
- 24次使用
-
- 知网AIGC检测服务系统
- 知网AIGC检测服务系统,专注于检测学术文本中的疑似AI生成内容。依托知网海量高质量文献资源,结合先进的“知识增强AIGC检测技术”,系统能够从语言模式和语义逻辑两方面精准识别AI生成内容,适用于学术研究、教育和企业领域,确保文本的真实性和原创性。
- 41次使用
-
- AIGC检测-Aibiye
- AIbiye官网推出的AIGC检测服务,专注于检测ChatGPT、Gemini、Claude等AIGC工具生成的文本,帮助用户确保论文的原创性和学术规范。支持txt和doc(x)格式,检测范围为论文正文,提供高准确性和便捷的用户体验。
- 38次使用
-
- 易笔AI论文
- 易笔AI论文平台提供自动写作、格式校对、查重检测等功能,支持多种学术领域的论文生成。价格优惠,界面友好,操作简便,适用于学术研究者、学生及论文辅导机构。
- 50次使用
-
- 笔启AI论文写作平台
- 笔启AI论文写作平台提供多类型论文生成服务,支持多语言写作,满足学术研究者、学生和职场人士的需求。平台采用AI 4.0版本,确保论文质量和原创性,并提供查重保障和隐私保护。
- 41次使用
查看更多
相关文章
-
- Flask框架安装技巧:让你的开发更高效
- 2024-01-03 501浏览
-
- Django框架中的并发处理技巧
- 2024-01-22 501浏览
-
- 提升Python包下载速度的方法——正确配置pip的国内源
- 2024-01-17 501浏览
-
- Python与C++:哪个编程语言更适合初学者?
- 2024-03-25 501浏览
-
- 品牌建设技巧
- 2024-04-06 501浏览