GPU 模式讲座 1 的笔记
来源:dev.to
2024-12-09 18:58:02
0浏览
收藏
在IT行业这个发展更新速度很快的行业,只有不停止的学习,才不会被行业所淘汰。如果你是文章学习者,那么本文《GPU 模式讲座 1 的笔记》就很适合你!本篇内容主要包括##content_title##,希望对大家的知识积累有所帮助,助力实战开发!
分析器
计算机性能取决于时间和内存的权衡。由于计算设备比较昂贵,所以大多数时候,时间是首先要关心的。
为什么要使用分析器?
- cuda 是异步的,因此无法使用 python 时间模块
- 分析器更加强大
工具
共有三个分析器:
- autograd 分析器:数值
- pytorch 分析器:视觉
- nvidia nsight 计算
autograd 分析器利用 torch.cuda.event() 来测量性能。
pytorch profiler 利用 profiler 上下文管理器 torch.profiler 中的 profile() 方法来分析性能。
您可以将结果导出为 .json 文件并将其上传到 chrome://tracing/ 进行可视化。
演示
课程提供了一个简单的程序来展示如何使用autograd profiler来分析三种平方运算方法的性能:
- 通过 torch.square()
- 由 ** 操作员
- 由 * 操作员
def time_pytorch_function(func, input): # cuda is async so can't use python time module start = torch.cuda.event(enable_timing=true) end = torch.cuda.event(enable_timing=true) # warmup for _ in range(5): func(input) start.record() func(input) end.record() torch.cuda.synchronize() return start.elapsed_time(end) time_pytorch_function(torch.square, b) time_pytorch_function(square_2, b) time_pytorch_function(square_3, b)
下面的结果是在 nvidia t4 gpu 上完成的。
profiling torch.square: self cpu time total: 10.577ms self cuda time total: 3.266ms profiling a * a: self cpu time total: 5.417ms self cuda time total: 3.276ms profiling a ** 2: self cpu time total: 6.183ms self cuda time total: 3.274ms
事实证明:
- cuda 运算速度比 cpu 更快。
- * 运算符执行的是 aten::multiply 操作,而不是 aten::pow,并且前者更快。这可能是因为乘法比 pow 使用得更多,并且许多开发人员花时间对其进行优化。
- cuda 上的性能差异很小。考虑到 cpu 时间,torch.square 是最慢的操作
- aten::square 是对 aten::pow 的调用
- 所有三种方法都启动了一个名为native::vectorized_elementwise_kernel<4的cuda内核,位于...
在 pytorch 中集成 cuda 内核
有几种方法可以做到这一点:
- 使用torch.utils.cpp_extendsion中的load_inline
- 使用 numba,它是一个编译器,可将经过修饰的 python 函数编译为在 cpu 和 gpu 上运行的机器代码
- 使用 triton
我们可以使用torch.utils.cpp_extendsion中的load_inline通过load_inline(name,cpp_sources,cuda_sources,functions,with_cuda,build_directory)将cuda内核加载为pytorch扩展。
from torch.utils.cpp_extension import load_inline square_matrix_extension = load_inline( name='square_matrix_extension', cpp_sources=cpp_source, cuda_sources=cuda_source, functions=['square_matrix'], with_cuda=true, extra_cuda_cflags=["-o2"], build_directory='./load_inline_cuda', # extra_cuda_cflags=['--expt-relaxed-constexpr'] ) a = torch.tensor([[1., 2., 3.], [4., 5., 6.]], device='cuda') print(square_matrix_extension.square_matrix(a))
动手实践
对均值操作使用 autograd 分析器
使用 autograd profiler 时,请记住:
- 录制前预热gpu,使gpu进入稳定状态
- 平均多次运行以获得更可靠的结果
import torch # method 1: use `torch.mean()` def mean_all_by_torch(input_tensor): return torch.mean(input_tensor) # method 2: use `mean()` of the tensor def mean_all_by_tensor(input_tensor): return input_tensor.mean() # method 3: use `torch.sum()` and `tensor.numel()` def mean_all_by_combination(input_tensor): return torch.sum(input_tensor) / input_tensor.numel() def time_pytorch_function(func, input_tensor, warmup=5, runs=100): # warmup for _ in range(warmup): func(input_tensor) times = [] start = torch.cuda.event(enable_timing=true) end = torch.cuda.event(enable_timing=true) for _ in range(runs): start.record() func(input_tensor) end.record() torch.cuda.synchronize() times.append(start.elapsed_time(end)) return sum(times) / len(times) input_tensor = torch.randn(10000, 10000).cuda() print("torch.mean() time:", time_pytorch_function(mean_all_by_torch, input_tensor)) print("tensor.mean() time:", time_pytorch_function(mean_all_by_tensor, input_tensor)) print("manual mean time:", time_pytorch_function(mean_all_by_combination, input_tensor)) with torch.profiler.profile() as prof: mean_all_by_torch(input_tensor) print(prof.key_averages().table(sort_by="cuda_time_total", row_limit=10)) with torch.profiler.profile() as prof: mean_all_by_tensor(input_tensor) print(prof.key_averages().table(sort_by="cuda_time_total", row_limit=10)) with torch.profiler.profile() as prof: mean_all_by_combination(input_tensor) print(prof.key_averages().table(sort_by="cuda_time_total", row_limit=10))
使用 pytorch 分析器进行均值操作
import torch from torch.profiler import profile, profileractivity with profile(activities=[profileractivity.cpu, profileractivity.cuda]) as prof: for _ in range(10): mean_tensor = torch.mean(torch.randn(10000, 10000).cuda()) prof.export_chrome_trace("mean_trace.json")
为 torch.mean() 实现 triton 代码
import triton import triton.language as tl import torch @triton.jit def mean_kernel( x_ptr, # pointer to input tensor output_ptr, # pointer to output tensor n_elements, # total number of elements BLOCK_SIZE: tl.constexpr, # number of elements per block ): pid = tl.program_id(0) block_start = pid * BLOCK_SIZE block_end = tl.minimum(block_start + BLOCK_SIZE, n_elements) acc = 0.0 for idx in range(block_start, block_end): x = tl.load(x_ptr + idx) acc += x block_mean = acc / n_elements # Store result tl.store(output_ptr + pid, block_mean) # Wrapper function def triton_mean(x: torch.Tensor) -> torch.Tensor: x = x.contiguous().view(-1) n_elements = x.numel() BLOCK_SIZE = 1024 grid = (triton.cdiv(n_elements, BLOCK_SIZE),) output = torch.empty(grid[0], device=x.device, dtype=x.dtype) mean_kernel[grid]( x_ptr=x, output_ptr=output, n_elements=n_elements, BLOCK_SIZE=BLOCK_SIZE, ) return output.sum() # Example usage: if __name__ == "__main__": # Create test tensor x = torch.randn(1000000, device='cuda') # Compare results torch_mean = torch.mean(x) triton_mean_result = triton_mean(x) print(f"PyTorch mean: {torch_mean}") print(f"Triton mean: {triton_mean_result}") print(f"Difference: {abs(torch_mean - triton_mean_result)}")
参考
- gpu 模式讲座 - github
- 活动 - pytorch
- pytorch 分析器
- nvidia nsight 计算
- torch.utils.cpp_extension.load_inline
- 海卫一
今天关于《GPU 模式讲座 1 的笔记》的内容介绍就到此结束,如果有什么疑问或者建议,可以在golang学习网公众号下多多回复交流;文中若有不正之处,也希望回复留言以告知!
版本声明
本文转载于:dev.to 如有侵犯,请联系study_golang@163.com删除
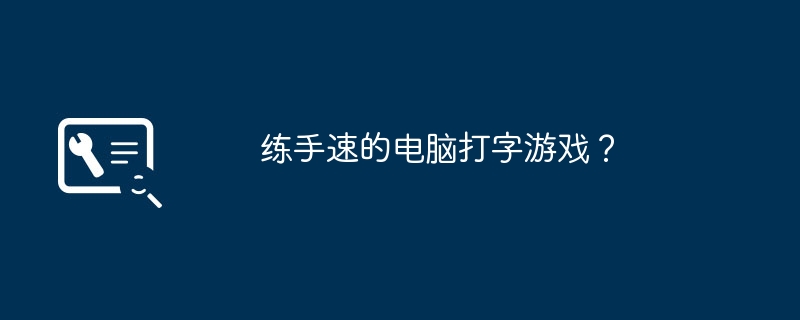
- 上一篇
- 练手速的电脑打字游戏?
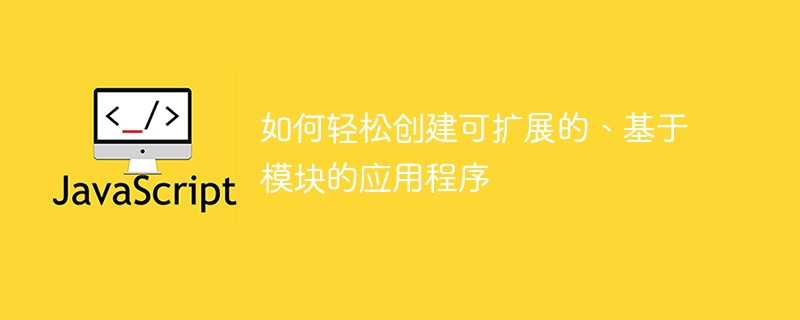
- 下一篇
- 如何轻松创建可扩展的、基于模块的应用程序
查看更多
最新文章
-
- 文章 · python教程 | 5分钟前 |
- Python中__slots__如何优化内存?
- 485浏览 收藏
-
- 文章 · python教程 | 1小时前 |
- Python热力图绘制教程及代码实战
- 191浏览 收藏
-
- 文章 · python教程 | 1小时前 | await unittest.IsolatedAsyncioTestCase asyncdef asyncio.gather AsyncMock
- Python异步代码测试技巧与实用方法
- 271浏览 收藏
-
- 文章 · python教程 | 1小时前 |
- VSCodePython开发环境配置:插件推荐与调试技巧
- 236浏览 收藏
-
- 文章 · python教程 | 2小时前 |
- VSCode配置Python:插件推荐与调试技巧
- 305浏览 收藏
-
- 文章 · python教程 | 2小时前 | Python 性能 代码可读性 装饰器模式 functools.wraps
- Python装饰器模式详解与实现教程
- 192浏览 收藏
-
- 文章 · python教程 | 2小时前 |
- Python爬虫数据存MySQL失败的解决方案
- 307浏览 收藏
查看更多
课程推荐
-
- 前端进阶之JavaScript设计模式
- 设计模式是开发人员在软件开发过程中面临一般问题时的解决方案,代表了最佳的实践。本课程的主打内容包括JS常见设计模式以及具体应用场景,打造一站式知识长龙服务,适合有JS基础的同学学习。
- 542次学习
-
- GO语言核心编程课程
- 本课程采用真实案例,全面具体可落地,从理论到实践,一步一步将GO核心编程技术、编程思想、底层实现融会贯通,使学习者贴近时代脉搏,做IT互联网时代的弄潮儿。
- 508次学习
-
- 简单聊聊mysql8与网络通信
- 如有问题加微信:Le-studyg;在课程中,我们将首先介绍MySQL8的新特性,包括性能优化、安全增强、新数据类型等,帮助学生快速熟悉MySQL8的最新功能。接着,我们将深入解析MySQL的网络通信机制,包括协议、连接管理、数据传输等,让
- 497次学习
-
- JavaScript正则表达式基础与实战
- 在任何一门编程语言中,正则表达式,都是一项重要的知识,它提供了高效的字符串匹配与捕获机制,可以极大的简化程序设计。
- 487次学习
-
- 从零制作响应式网站—Grid布局
- 本系列教程将展示从零制作一个假想的网络科技公司官网,分为导航,轮播,关于我们,成功案例,服务流程,团队介绍,数据部分,公司动态,底部信息等内容区块。网站整体采用CSSGrid布局,支持响应式,有流畅过渡和展现动画。
- 484次学习
查看更多
AI推荐
-
- AI Make Song
- AI Make Song是一款革命性的AI音乐生成平台,提供文本和歌词转音乐的双模式输入,支持多语言及商业友好版权体系。无论你是音乐爱好者、内容创作者还是广告从业者,都能在这里实现“用文字创造音乐”的梦想。平台已生成超百万首原创音乐,覆盖全球20个国家,用户满意度高达95%。
- 20次使用
-
- SongGenerator
- 探索SongGenerator.io,零门槛、全免费的AI音乐生成器。无需注册,通过简单文本输入即可生成多风格音乐,适用于内容创作者、音乐爱好者和教育工作者。日均生成量超10万次,全球50国家用户信赖。
- 16次使用
-
- BeArt AI换脸
- 探索BeArt AI换脸工具,免费在线使用,无需下载软件,即可对照片、视频和GIF进行高质量换脸。体验快速、流畅、无水印的换脸效果,适用于娱乐创作、影视制作、广告营销等多种场景。
- 16次使用
-
- 协启动
- SEO摘要协启动(XieQiDong Chatbot)是由深圳协启动传媒有限公司运营的AI智能服务平台,提供多模型支持的对话服务、文档处理和图像生成工具,旨在提升用户内容创作与信息处理效率。平台支持订阅制付费,适合个人及企业用户,满足日常聊天、文案生成、学习辅助等需求。
- 19次使用
-
- Brev AI
- 探索Brev AI,一个无需注册即可免费使用的AI音乐创作平台,提供多功能工具如音乐生成、去人声、歌词创作等,适用于内容创作、商业配乐和个人创作,满足您的音乐需求。
- 21次使用
查看更多
相关文章
-
- Flask框架安装技巧:让你的开发更高效
- 2024-01-03 501浏览
-
- Django框架中的并发处理技巧
- 2024-01-22 501浏览
-
- 提升Python包下载速度的方法——正确配置pip的国内源
- 2024-01-17 501浏览
-
- Python与C++:哪个编程语言更适合初学者?
- 2024-03-25 501浏览
-
- 品牌建设技巧
- 2024-04-06 501浏览