使用 SLM 从头开始构建 ReAct Agent
今天golang学习网给大家带来了《使用 SLM 从头开始构建 ReAct Agent》,其中涉及到的知识点包括等等,无论你是小白还是老手,都适合看一看哦~有好的建议也欢迎大家在评论留言,若是看完有所收获,也希望大家能多多点赞支持呀!一起加油学习~
在这篇文章中,我将演示如何使用小语言模型 (slm) 创建函数调用代理。利用 slm 可以带来一系列好处,特别是与 lora 适配器等工具配合使用时,可以实现高效的微调和执行。虽然大型语言模型 (llm) 功能强大,但它们可能会占用大量资源且速度缓慢。另一方面,slm 更加轻量级,使其非常适合硬件资源有限的环境或低延迟至关重要的特定用例。
通过将slm与lora适配器结合使用,我们可以将推理和函数执行任务分开以优化性能。例如,模型可以使用适配器执行复杂的函数调用,并在没有适配器的情况下处理推理或思考任务,从而节省内存并提高速度。这种灵活性非常适合构建函数调用代理等应用程序,而无需大型模型所需的基础设施。
此外,slm 可以轻松扩展以在计算能力有限的设备上运行,这使其成为优先考虑成本和效率的生产环境的理想选择。在此示例中,我们将使用通过 unsloth 在 salesforce/xlam-function-calling-60k 数据集上训练的自定义模型,演示如何利用 slm 创建高性能、低资源的 ai 应用程序.
此外,这里讨论的方法可以扩展到更强大的模型,例如llama 3.1-8b,它具有内置的函数调用功能,在需要更大的模型时提供平滑的过渡。
1. 使用 unsloth 启动模型和分词器
我们首先使用 unsloth 设置模型和标记器。在这里,我们定义最大序列长度为 2048,尽管这个长度是可以调整的。我们还启用4 位量化来减少内存使用量,非常适合在内存较低的硬件上运行模型。
from unsloth import fastlanguagemodel max_seq_length = 2048 # choose any! we auto support rope scaling internally! dtype = none # none for auto detection. float16 for tesla t4, v100, bfloat16 for ampere+ load_in_4bit = true # use 4bit quantization to reduce memory usage. can be false. model, tokenizer = fastlanguagemodel.from_pretrained( model_name = "akshayballal/phi-3.5-mini-xlam-function-calling", max_seq_length = max_seq_length, dtype = dtype, load_in_4bit = load_in_4bit, ) fastlanguagemodel.for_inference(model);
2. 实施受控发电的停止标准
为了确保代理在函数调用后暂停执行,我们定义了停止条件。当模型输出关键字“pause”时,这将停止生成,从而允许代理获取函数调用的结果。
from transformers import stoppingcriteria, stoppingcriterialist import torch class keywordsstoppingcriteria(stoppingcriteria): def __init__(self, keywords_ids:list): self.keywords = keywords_ids def __call__(self, input_ids: torch.longtensor, _: torch.floattensor, **kwargs) -> bool: if input_ids[0][-1] in self.keywords: return true return false stop_ids = [17171] stop_criteria = keywordsstoppingcriteria(stop_ids)
3. 定义函数调用工具
接下来,我们定义代理在执行期间将使用的函数。这些python函数将充当代理可以调用的“工具”。返回类型必须明确,并且函数应包含描述性文档字符串,因为代理将依赖于此来选择正确的工具。
def add_numbers(a: int, b: int) -> int: """ this function takes two integers and returns their sum. parameters: a (int): the first integer to add. b (int): the second integer to add. """ return a + b def square_number(a: int) -> int: """ this function takes an integer and returns its square. parameters: a (int): the integer to be squared. """ return a * a def square_root_number(a: int) -> int: """ this function takes an integer and returns its square root. parameters: a (int): the integer to calculate the square root of. """ return a ** 0.5
4. 为代理生成工具描述
这些函数描述将被构造成一个字典列表。代理将使用这些来了解可用的工具及其参数。
tool_descriptions = [] for tool in tools: spec = { "name": tool.__name__, "description": tool.__doc__.strip(), "parameters": [ { "name": param, "type": arg.__name__ if hasattr(arg, '__name__') else str(arg), } for param, arg in tool.__annotations__.items() if param != 'return' ] } tool_descriptions.append(spec) tool_descriptions
这就是输出的样子
[{'name': 'add_numbers', 'description': 'this function takes two integers and returns their sum.\n\n parameters:\n a (int): the first integer to add.\n b (int): the second integer to add.', 'parameters': [{'name': 'a', 'type': 'int'}, {'name': 'b', 'type': 'int'}]}, {'name': 'square_number', 'description': 'this function takes an integer and returns its square.\n\n parameters:\n a (int): the integer to be squared.', 'parameters': [{'name': 'a', 'type': 'int'}]}, {'name': 'square_root_number', 'description': 'this function takes an integer and returns its square root.\n\n parameters:\n a (int): the integer to calculate the square root of.', 'parameters': [{'name': 'a', 'type': 'int'}]}]
5. 创建代理类
然后我们创建代理类,它将系统提示、函数调用提示、工具和消息作为输入,并返回代理的响应。
- __call__ 是使用消息调用代理时调用的函数。它将消息添加到消息列表并返回代理的响应。
- execute 是被调用以生成代理响应的函数。它使用模型来生成响应。
- function_call 是被调用以生成代理响应的函数。它使用函数调用模型来生成响应。
import ast class agent: def __init__( self, system: str = "", function_calling_prompt: str = "", tools=[] ) -> none: self.system = system self.tools = tools self.function_calling_prompt = function_calling_prompt self.messages: list = [] if self.system: self.messages.append({"role": "system", "content": system}) def __call__(self, message=""): if message: self.messages.append({"role": "user", "content": message}) result = self.execute() self.messages.append({"role": "assistant", "content": result}) return result def execute(self): with model.disable_adapter(): # disable the adapter for thinking and reasoning inputs = tokenizer.apply_chat_template( self.messages, tokenize=true, add_generation_prompt=true, return_tensors="pt", ) output = model.generate( input_ids=inputs, max_new_tokens=128, stopping_criteria=stoppingcriterialist([stop_criteria]), ) return tokenizer.decode( output[0][inputs.shape[-1] :], skip_special_tokens=true ) def function_call(self, message): inputs = tokenizer.apply_chat_template( [ { "role": "user", "content": self.function_calling_prompt.format( tool_descriptions=tool_descriptions, query=message ), } ], tokenize=true, add_generation_prompt=true, return_tensors="pt", ) output = model.generate(input_ids=inputs, max_new_tokens=128, temperature=0.0) prompt_length = inputs.shape[-1] answer = ast.literal_eval( tokenizer.decode(output[0][prompt_length:], skip_special_tokens=true) )[ 0 ] # get the output of the function call model as a dictionary print(answer) tool_output = self.run_tool(answer["name"], **answer["arguments"]) return tool_output def run_tool(self, name, *args, **kwargs): for tool in self.tools: if tool.__name__ == name: return tool(*args, **kwargs)
6. 定义系统和函数调用提示
我们现在定义两个关键提示:
- 系统提示:智能体推理和工具使用的核心逻辑,遵循react模式。
- 函数调用提示:通过传递相关工具描述和查询来启用函数调用。
system_prompt = f""" you run in a loop of thought, action, pause, observation. at the end of the loop you output an answer use thought to describe your thoughts about the question you have been asked. use action to run one of the actions available to you - then return pause. observation will be the result of running those actions. stop when you have the answer. your available actions are: {tools} example session: question: what is the mass of earth times 2? thought: i need to find the mass of earth action: get_planet_mass: earth pause observation: 5.972e24 thought: i need to multiply this by 2 action: calculate: 5.972e24 * 2 pause observation: 1,1944×10e25 if you have the answer, output it as the answer. answer: \\{{1,1944×10e25\\}}. pause now it's your turn: """.strip() function_calling_prompt = """ you are a helpful assistant. below are the tools that you have access to. \n\n### tools: \n{tool_descriptions} \n\n### query: \n{query} \n """
7. 实现react循环
最后,我们定义了一个循环,使代理能够与用户交互,执行必要的函数调用,并返回正确的答案。
import re def loop_agent(agent: Agent, question, max_iterations=5): next_prompt = question i = 0 while i < max_iterations: result = agent(next_prompt) print(result) if "Answer:" in result: return result action = re.findall(r"Action: (.*)", result) if action: tool_output= agent.function_call(action) next_prompt = f"Observation: {tool_output}" print(next_prompt) else: next_prompt = "Observation: tool not found" i += 1 return result agent = Agent( system=system_prompt, function_calling_prompt=function_calling_prompt, tools=tools) loop_agent(agent, "what is the square root of the difference between 32^2 and 54");
结论
按照此分步指南,您可以使用使用 unsloth 和 lora 适配器 训练的自定义模型创建函数调用代理。这种方法确保高效的内存使用,同时保持强大的推理和函数执行能力。
通过将此方法扩展到更大的模型或自定义代理可用的功能来进一步探索。
以上就是本文的全部内容了,是否有顺利帮助你解决问题?若是能给你带来学习上的帮助,请大家多多支持golang学习网!更多关于文章的相关知识,也可关注golang学习网公众号。
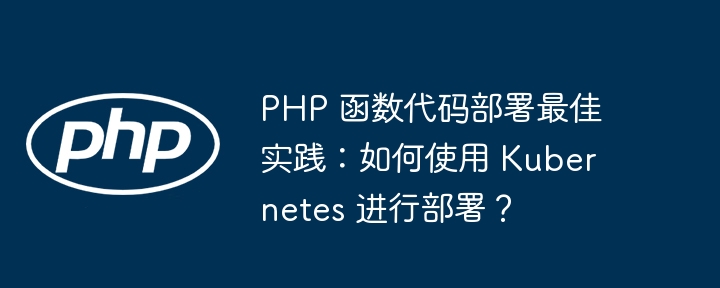
- 上一篇
- PHP 函数代码部署最佳实践:如何使用 Kubernetes 进行部署?
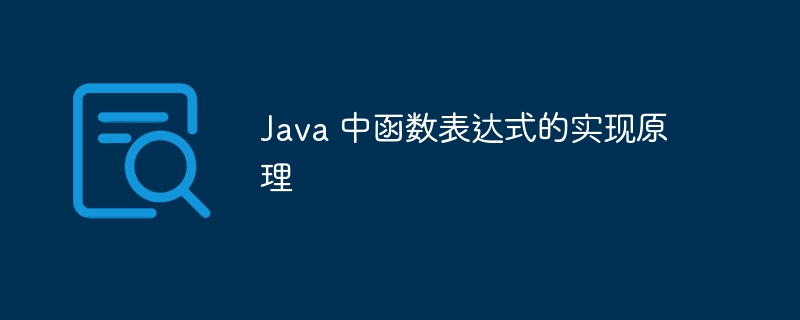
- 下一篇
- Java 中函数表达式的实现原理
-
- 文章 · python教程 | 5小时前 |
- Python正则匹配Unicode字符技巧
- 346浏览 收藏
-
- 文章 · python教程 | 5小时前 |
- Python中lambda的用法及匿名函数创建方法
- 127浏览 收藏
-
- 文章 · python教程 | 5小时前 |
- PyCharm运行代码方法详解
- 372浏览 收藏
-
- 文章 · python教程 | 6小时前 |
- Python中eval的作用与使用详解
- 244浏览 收藏
-
- 文章 · python教程 | 6小时前 |
- 正则表达式量词有哪些及用法详解
- 391浏览 收藏
-
- 文章 · python教程 | 7小时前 | Python 链式比较
- Python链式比较技巧,简化条件判断方法
- 225浏览 收藏
-
- 文章 · python教程 | 7小时前 |
- PyCharm解释器选择指南与建议
- 174浏览 收藏
-
- 文章 · python教程 | 7小时前 |
- 模块导入错误:ImportError与ModuleNotFoundError区别详解
- 339浏览 收藏
-
- 文章 · python教程 | 7小时前 |
- PyCharm中文界面设置教程3步搞定
- 317浏览 收藏
-
- 文章 · python教程 | 7小时前 |
- Python分页实现与优化技巧分享
- 384浏览 收藏
-
- 文章 · python教程 | 7小时前 |
- Pythonunittest使用教程详解
- 139浏览 收藏
-
- 文章 · python教程 | 7小时前 |
- Python类型注解教程与实用技巧
- 463浏览 收藏
-
- 前端进阶之JavaScript设计模式
- 设计模式是开发人员在软件开发过程中面临一般问题时的解决方案,代表了最佳的实践。本课程的主打内容包括JS常见设计模式以及具体应用场景,打造一站式知识长龙服务,适合有JS基础的同学学习。
- 542次学习
-
- GO语言核心编程课程
- 本课程采用真实案例,全面具体可落地,从理论到实践,一步一步将GO核心编程技术、编程思想、底层实现融会贯通,使学习者贴近时代脉搏,做IT互联网时代的弄潮儿。
- 508次学习
-
- 简单聊聊mysql8与网络通信
- 如有问题加微信:Le-studyg;在课程中,我们将首先介绍MySQL8的新特性,包括性能优化、安全增强、新数据类型等,帮助学生快速熟悉MySQL8的最新功能。接着,我们将深入解析MySQL的网络通信机制,包括协议、连接管理、数据传输等,让
- 497次学习
-
- JavaScript正则表达式基础与实战
- 在任何一门编程语言中,正则表达式,都是一项重要的知识,它提供了高效的字符串匹配与捕获机制,可以极大的简化程序设计。
- 487次学习
-
- 从零制作响应式网站—Grid布局
- 本系列教程将展示从零制作一个假想的网络科技公司官网,分为导航,轮播,关于我们,成功案例,服务流程,团队介绍,数据部分,公司动态,底部信息等内容区块。网站整体采用CSSGrid布局,支持响应式,有流畅过渡和展现动画。
- 484次学习
-
- 免费AI认证证书
- 科大讯飞AI大学堂推出免费大模型工程师认证,助力您掌握AI技能,提升职场竞争力。体系化学习,实战项目,权威认证,助您成为企业级大模型应用人才。
- 12次使用
-
- 茅茅虫AIGC检测
- 茅茅虫AIGC检测,湖南茅茅虫科技有限公司倾力打造,运用NLP技术精准识别AI生成文本,提供论文、专著等学术文本的AIGC检测服务。支持多种格式,生成可视化报告,保障您的学术诚信和内容质量。
- 157次使用
-
- 赛林匹克平台(Challympics)
- 探索赛林匹克平台Challympics,一个聚焦人工智能、算力算法、量子计算等前沿技术的赛事聚合平台。连接产学研用,助力科技创新与产业升级。
- 187次使用
-
- 笔格AIPPT
- SEO 笔格AIPPT是135编辑器推出的AI智能PPT制作平台,依托DeepSeek大模型,实现智能大纲生成、一键PPT生成、AI文字优化、图像生成等功能。免费试用,提升PPT制作效率,适用于商务演示、教育培训等多种场景。
- 174次使用
-
- 稿定PPT
- 告别PPT制作难题!稿定PPT提供海量模板、AI智能生成、在线协作,助您轻松制作专业演示文稿。职场办公、教育学习、企业服务全覆盖,降本增效,释放创意!
- 161次使用
-
- Flask框架安装技巧:让你的开发更高效
- 2024-01-03 501浏览
-
- Django框架中的并发处理技巧
- 2024-01-22 501浏览
-
- 提升Python包下载速度的方法——正确配置pip的国内源
- 2024-01-17 501浏览
-
- Python与C++:哪个编程语言更适合初学者?
- 2024-03-25 501浏览
-
- 品牌建设技巧
- 2024-04-06 501浏览